If you are trying to reach FDAnews.com, you’re in the right place.
We regret to inform you the production of FDAnews publications and databases has come to an end and we are closing our doors. Thank you for your support and readership over the years.
If you have any questions specific to legacy FDAnews publications, please email customerservice@fdanews.com.
If you are looking for clinical research information and insights, or information about clinical trials, we invite you to visit:
- Avoca Quality Consortium (AQC) a pre-competitive collaborative consortium of 200+ companies dedicated to elevating clinical trial quality and unlocking resources to improve clinical research.
- WCG Insights to access some of the latest thought leadership relevant to the clinical research industry.
- Clinical Trial Listing Service to find details about current clinical trial opportunities.
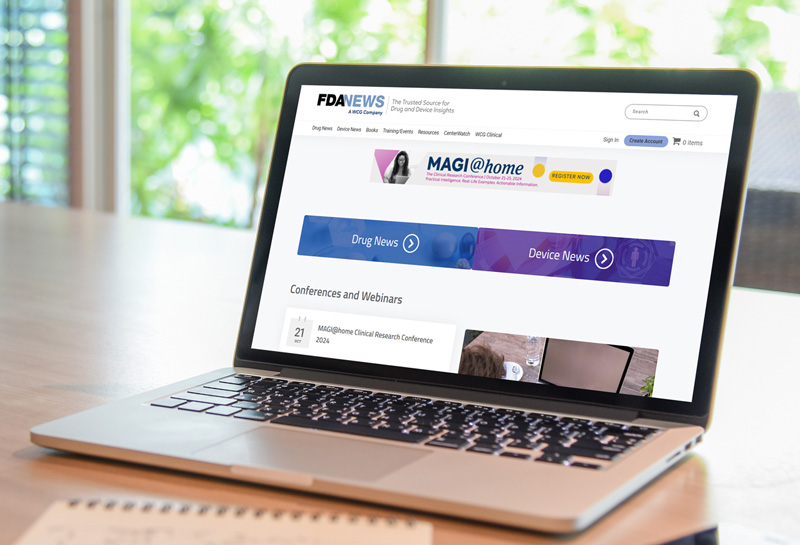
About the Avoca Quality Consortium
The Avoca Quality Consortium is a pre-competitive collaborative of sponsors, providers, CROs, and sites who share the objective of elevating clinical trial quality and building efficiencies into clinical trial execution, while bringing key stakeholders into greater alignment. Gain access to a comprehensive library of leading practices and resources across the entire clinical trial ecosystem, including medical device, inspection readiness, provider qualification/oversight, QbD/RBQM, and more.